A Symposium on Communication & Intelligence
October 18th, 2024, 9:00 AM - 5:30 PM
JCL 390 (John Crerar Library)
This symposium is a place to discuss how to think about the intertwining of Communication and Intelligence in an age of machines that talk back. We increasingly interact with technology that attempts (simulates?) communication with humans, rather than just signaling information passively for a user to interpret. While the term “AI” has been around since the 50’s, the explosion of products, services, and bots that create a complex dialogue with their users (and even among themselves!) has created a phase shift in how we interact with machines that wield language. How should we study AI that communicates, rather than just solves a well-defined problem on its own? What are the structures of the new communication networks AI helps create, spanning people, machines, and institutions? What “counts” as communication and can AI help us decompose how it works more clearly? Can machines that communicate help us communicate better? Or are they merely eroding and biasing our human communication by polluting the commons? In this symposium we will discuss how communication and intelligence interact—in humans, in machines, and between them.
Invited Speakers
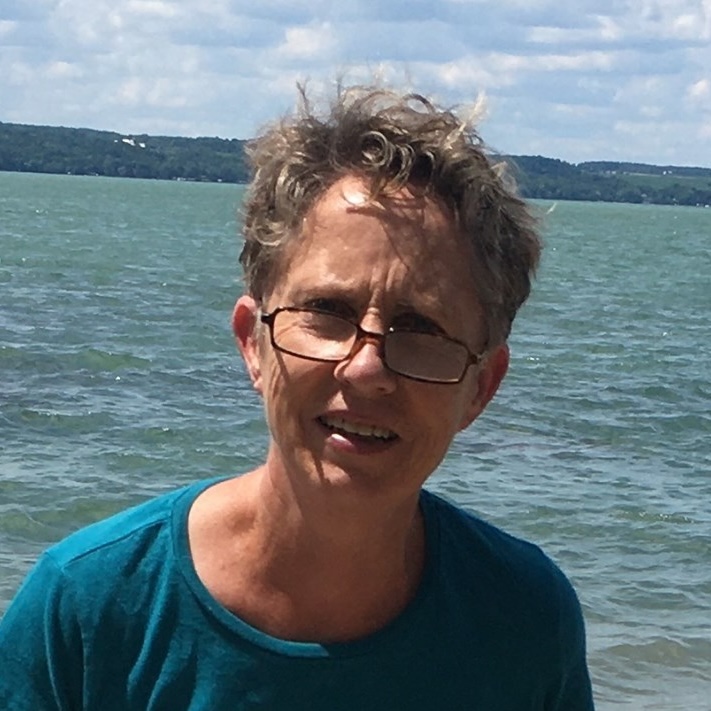
Claire Cardie
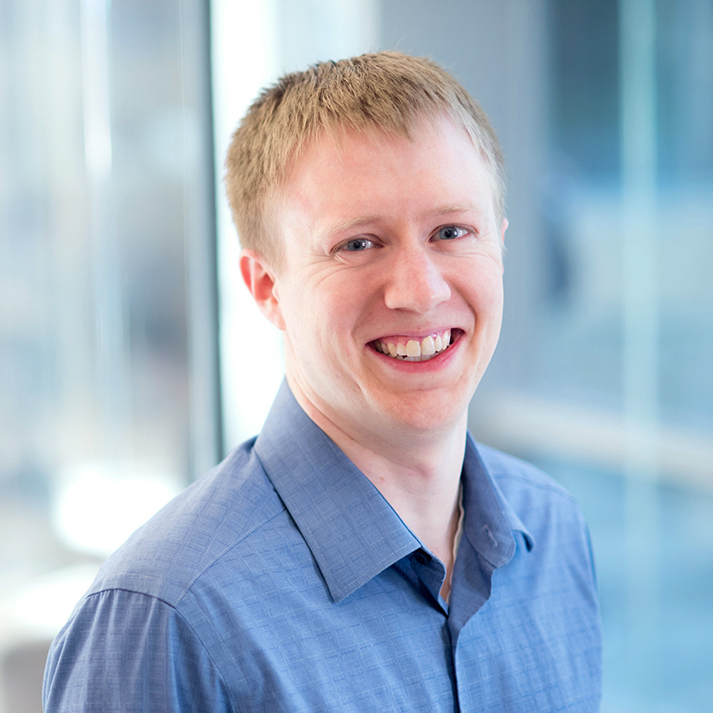
Greg Durrett
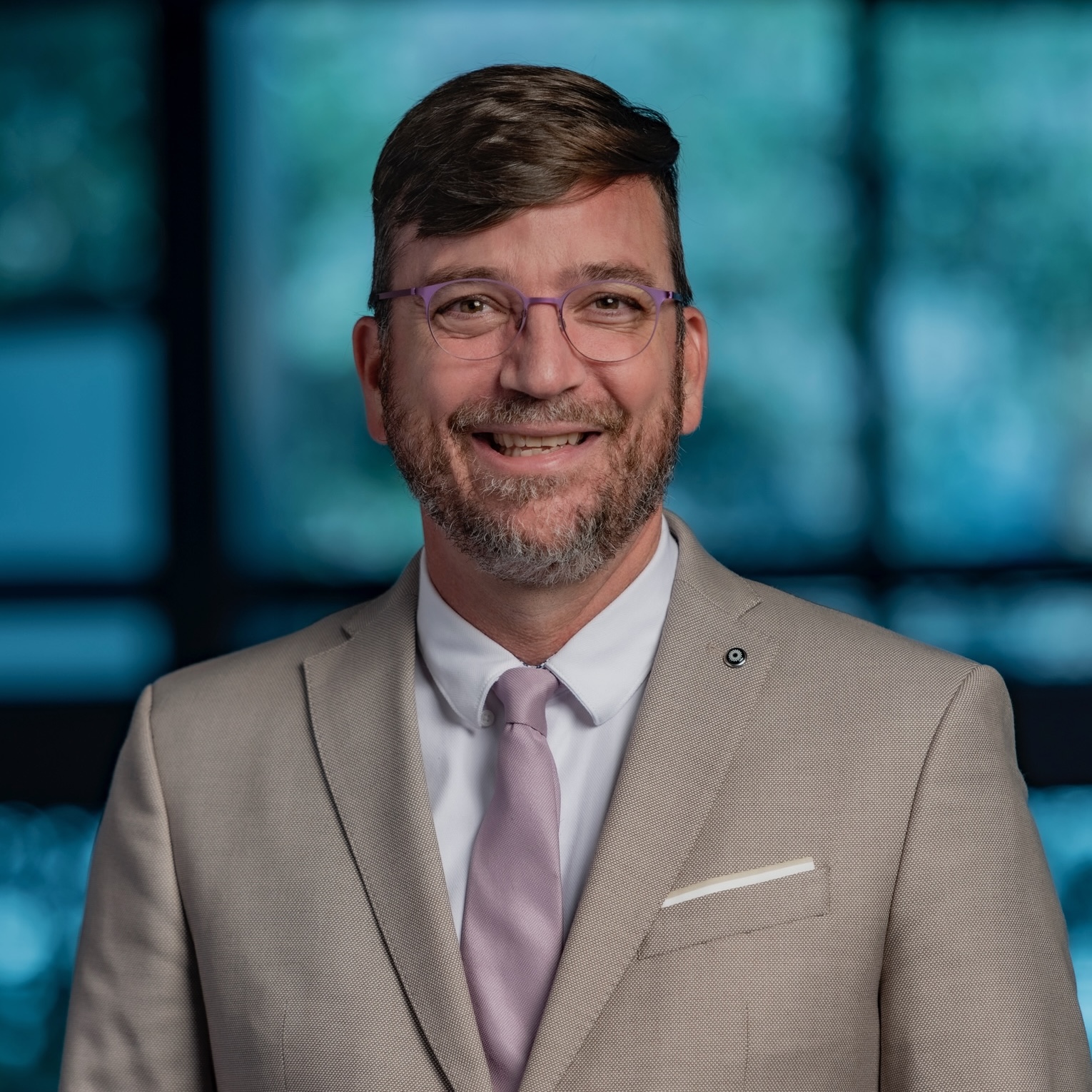
Chris Callison-Burch
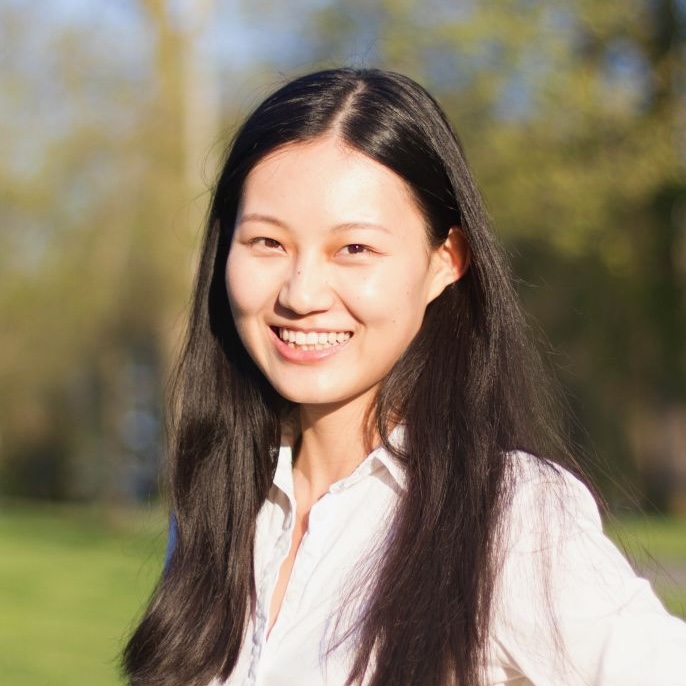
He He
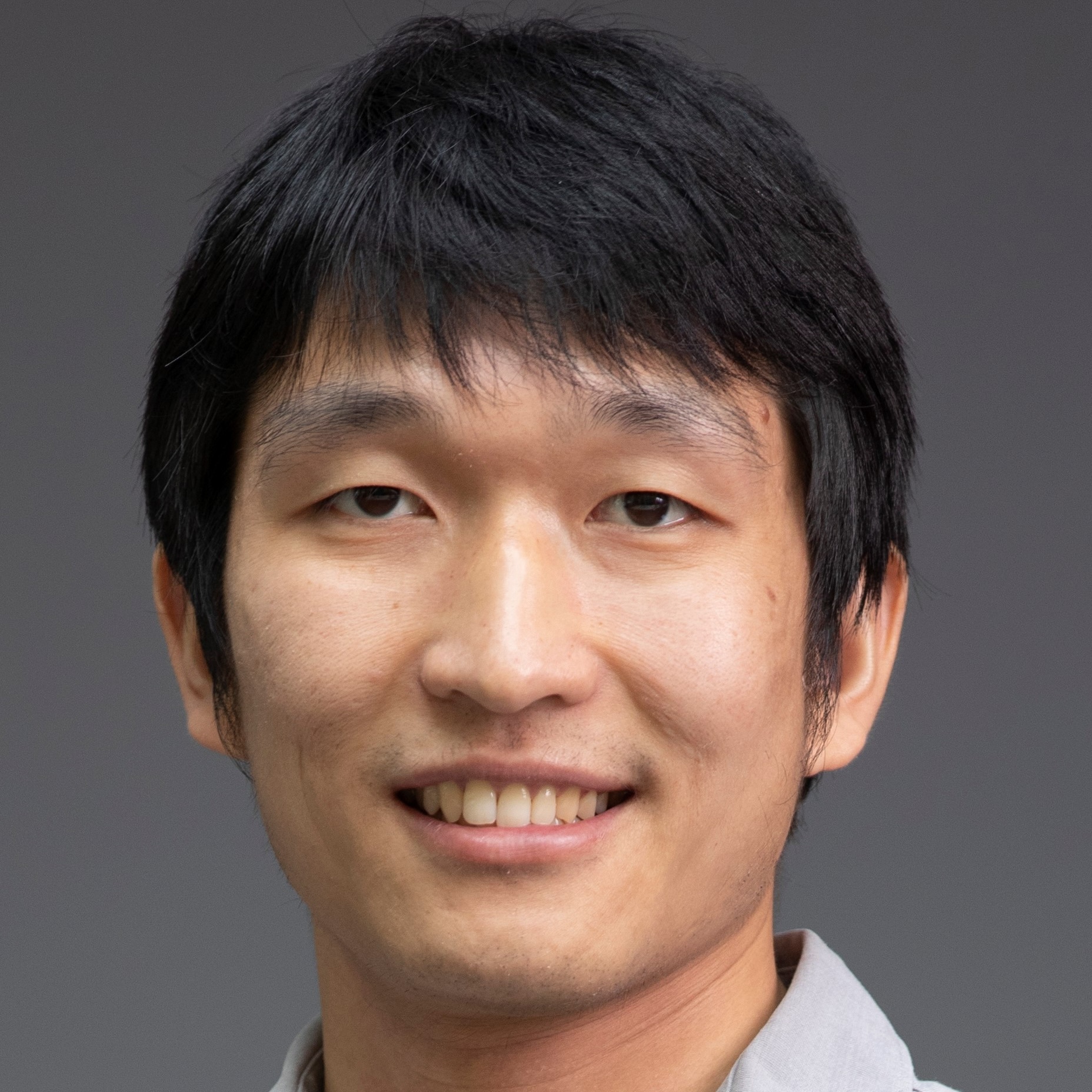
Chi Wang
Call for poster presentations
We invite all researchers and practitioners to submit poster presentations for the Symposium on Communication & Intelligence. This is an opportunity to showcase your work, share insights, and engage in discussions about the intersection of AI and human communication. We are particularly interested in presentations that examine opportunities and challenges to achieve complementary and beneficent AI. Automation will happen inevitably—but where are the opportunities for symbiosis? Poster presenters will have the opportunity to display their posters at the Symposium and engage with fellow attendees during poster sessions. This is a chance to receive feedback, establish collaborations, and contribute to meaningful conversations about the future of interaction between humans and AI. Please submit your abstract here by October 5th.
Organization
The organizing committee for the Sympoisum on Communication & Intelligence are Chenhao Tan and Ari Holtzman. This event is made possible by generous support of the Stevanovich Center for Financial Mathematics.
Schedule
Abstract
What are future AI applications like and how do we empower every developer to build them? This talk will discuss the trend of agentic AI and the core design considerations of agentic AI programming frameworks. It will then dive into a pioneering attempt, AutoGen, covering the key concepts, how it is applied across a broad spectrum of tasks and industries, and recent research progress. It ends with open questions.
Speaker Bio
Chi is the founder of AutoGen, a popular and rapidly growing open-source framework for agentic AI, with multiple awards such as best paper of ICLR’24 LLM Agents Workshop, Open100, and TheSequence’s pick of 5 favorite AI papers in 2023. His work is covered by Forbes, The Economist, WIRED etc. Chi is also the founder of FLAML, a fast open-source library for AutoML & tuning used widely by Microsoft, Google, Amazon, Databricks etc. Chi has 15+ years of research experience in Computer Science and work experience in Google DeepMind, Microsoft Research and Meta. Chi has a PhD in Computer Science from University of Illinois at Urbana-Champaign (with a SIGKDD Data Science/Data Mining PhD Dissertation Award), and a BS in Computer Science from Tsinghua University.
Abstract
Teaching machines to comprehend textual information has been a challenging and long-standing goal for research in natural language processing (NLP). Within the paradigm of machine learning for NLP the field has studied a multitude of linguistic phenomena and developed methods for tackling a wide range of language technology applications. The recent advent of large language models (LLMs), however, has fundamentally changed research trajectories in the field: many of the tasks that we were working on were effectively “solved” by very large transformer-based models trained on massive amounts of online text. This talk will present work on three problems that still cause problems for LLMs. Perhaps surprisingly, these problems are not new, but come from long-studied subareas of NLP — question answering, abductive reasoning, and information extraction.
Speaker Bio
Claire Cardie is the John C. Ford Professor of Engineering in the Departments of Computer Science and Information Science at Cornell University. She has worked since the early 1990’s on the application of machine learning methods to problems in Natural Language Processing — on topics ranging from information extraction, noun phrase coreference resolution, text summarization and question answering to the automatic analysis of opinions, argumentation, and deception in text. She has been Program Chair for ACL/COLING, EMNLP and CoNLL, and General Chair for ACL in 2018. Cardie is an ACL Fellow, a AAAI Fellow, an ACM Fellow, and a Fellow of the American Association for the Advancement of Science (AAAS). At Cornell, she led the development of the university’s academic programs in Information Science, was the founding Chair of its Information Science Department, and is currently serving as the inaugural Associate Dean for Education in Cornell’s Ann S. Bowers College of Computing and Information Science.
Abstract
Proponents of LLM scaling assert that training a giant model on as much data as possible can eventually solve most language tasks, perhaps even leading to AGI. However, frontier LLMs still fall short on complex problems in long-tail domains. Errors occur somewhere in the process of encoding the necessary knowledge, surfacing it for a specific prompt, and synthesizing it with other input data. In this talk, I will argue that specialization is the right approach to improve LLMs here; that is, modifying them through training or other means to improve their factuality and reasoning capabilities. First, I will show that specialization is necessary: inference-only approaches like chain-of-thought prompting are not sufficient. Second, I will present our fact-checking system MiniCheck, which is fine-tuned on specialized data to detect factual errors in LLM responses, leading to a better detector than frontier models like GPT-4. Finally, I will discuss how to specialize LLMs to be better at logical reasoning. I argue that we need (a) better fine-tuning methods which make targeted adjustments to model behavior; (b) improved inference capabilities, such as a differentiable theorem prover that can be plugged into a standard Transformer. These forms of specialization represent a path towards fundamentally new capabilities in factuality and reasoning beyond what can be achieved in current models.
Speaker Bio
Greg Durrett is an associate professor of Computer Science at UT Austin. He received his BS in Computer Science and Mathematics from MIT and his PhD in Computer Science from UC Berkeley, where he was advised by Dan Klein. His research is broadly in the areas of natural language processing and machine learning. Currently, his group’s focus is on techniques for reasoning about knowledge in text, verifying factuality of LLM generations, and building systems using LLMs as primitives. He is a 2023 Sloan Research Fellow and a recipient of a 2022 NSF CAREER award. He has co-organized the Workshop on Natural Language Reasoning and Structured Explanations at ACL 2023 and ACL 2024, as well as workshops on low-resource NLP and NLP for programming. He has served in numerous roles for *CL conferences, including as a member of the NAACL Board since 2024.
Abstract
This presentation discusses research on using large language models (LLMs) to build explainable classifiers. It will show off work from my PhD students and collaborators on several recent research directions:
- Image classification with explainable features (arxiv.org/abs/2211.11158)
- Text classification with explainable features (work in progress)
- The importance of faithfulness in explanations (arxiv.org/abs/2209.11326)
- A faithful “chain of thought” LLM reasoner that produces code in its explanations (arxiv.org/abs/2301.13379)
The talk will cover joint work with: Adam Stein, Ajay Patel, Ansh Kothary, Artemis Panagopoulou, Daniel Jin, Delip Rao, Eric Wong, Harry Li Zhang, Kathleen McKeown, Marianna Apidianaki, Mark Yatskar, Shenghao Zhou, Shreya Havaldar, Veronica Qing Lyu, Yue Yang, and others.
Speaker Bio
Chris Callison-Burch is a Professor of Computer and Information Science at the University of Pennsylvania. His course on Artificial Intelligence has one of the highest enrollments at the university with over 500 students taking the class each Fall. He is best known for his research into natural language processing. His current research is focused on applications of large language models to long-standing challenges in artificial intelligence. His PhD students joke that now whenever they ask him anything his first response is “Have you tried GPT for that?” Prof Callison-Burch has more than 150 publications, which have been cited over 25,000 times. He is a Sloan Research Fellow, and he has received faculty research awards from Google, Microsoft, Amazon, Facebook, and Roblox, in addition to funding from DARPA, IARPA, and the NSF.
Abstract
The alignment of large language models (LLMs) with human values is increasingly guided by human feedback, but this process is not without risks. In this talk, I will explore two critical aspects of human-in-the-loop training for LLMs. First, I will show how reinforcement learning from human feedback (RLHF) unintentionally teaches models to be more persuasive rather than accurate, making it difficult for humans to verify their answers. Second, I will show that post-training potentially dilutes diverse preferences of various human groups. Together, these two results underscore the importance of rethinking how and from whom we collect feedback to ensure robust and fair AI systems.